Reliability-based global path planning incorporates reliability constraints into path planning to ensure that off-road autonomous ground vehicles can operate reliably in uncertain off-road environments. Current two-stage reliability-based path planning methods involve separate stages for surrogate modeling of mobility prediction and global path planning, resulting in a large number of unnecessary mobility simulations that makes the approaches computationally expensive. To tackle this challenge, this work proposes a novel efficient reliability-based global path planning approach, named ER-RRT*, which couples adaptive surrogate modeling with the rapidly-exploring random tree star (RRT*) algorithm. Firstly, a surrogate model for vehicle mobility prediction is used to guide the exploration of random trees subject to a mobility reliability constraint. Subsequently, the exploration trees and reliability assessment are employed to inform mobility simulations for the surrogate model refinement. These steps are implemented iteratively and thereby drastically reducing the required mobility simulations for path planning through the integration of adaptive surrogate modeling with global path planning. With a focus on the uncertainty in the slope map and soil properties of deformable terrain, we demonstrate ER-RRT* using a case study and compare it with the current two-stage approach. The results show that ER-RRT* is much more efficient than the current method in both computational time and the required number of mobility simulations for surrogate model construction. In addition, the path identified by ER-RRT* exhibits a comparable cost in distance to its counterpart obtained using the two-stage method.
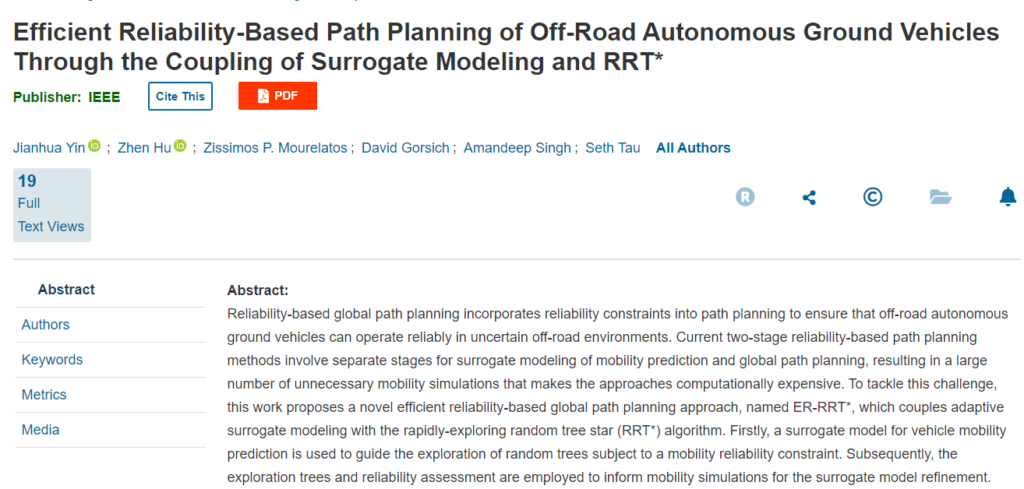